AI has emerged as a business force of its own kind, but its potential remains largely untapped for many organizations struggling with real-world implementation.
However, realizing AI’s potential isn’t without its challenges. Businesses often face hurdles like data readiness, alignment of AI initiatives with business goals, and gaps in technical skills—all of which can stall progress and lead to POC “purgatory.”
Addressing these barriers head-on empowers organizations to overcome the hype and deliver true AI value, aligning each deployment with both technological feasibility and strategic objectives.
In brief:
- The success of AI initiatives in companies depends on having the right kind of data, realistic approach to adoption within the context of business and clear, measurable objectives.
- GenAI will soon go from language processing, information synthesis and creative and adapting problem-solving, to autonomous task completion, adaptive learning and skills acquisition and collaborative problem solving.
- Key areas of creating value with AI lay in: information access and knowledge process automation, AI-assisted software development, sales and marketing optimization, support and customer care automation, legal automation and compliance monitoring.
Why Most AI Initiatives Fail to Deliver Value
AI projects often start with enthusiasm and promise but stumble along the way, failing to deliver tangible results.
Companies rush in, spurred by the hype around AI, but soon face obstacles that turn their excitement into disillusionment. Here’s why so many AI initiatives fall short of expectations—and how to address these challenges.
The Hype vs. Reality of AI in Business
It’s easy to get swept up in the excitement around AI. High-profile success stories and media coverage paint a picture of a technology that can transform any business overnight. This buzz often pushes companies to jump in without fully understanding what AI can or can’t do for them. But AI isn’t a one-size-fits-all solution, and the real-world complexities quickly surface.
For instance, while generative AI overview tools like ChatGPT can create human-like text or solve specific queries, they don’t inherently understand a company’s unique context or data. Tribe AI’s experience shows that AI’s effectiveness depends on:
- Clear objectives
- The right data
- Realistic approach
Most Common Pitfalls in AI Implementation
When companies launch AI initiatives, they often encounter roadblocks that they didn’t anticipate. Here are some of the most common pitfalls:
- Lack of data readiness: AI thrives on data, but not all data is equal. Often, companies lack the clean, structured data that AI requires, or their data is scattered across silos, making it difficult to harness. Data readiness from the start, ensuring that the AI model has quality information to work with, is the correct approach that needs to be implemented from the get-go.
- Inadequate technical expertise: AI is a specialized field. Many organizations don’t have the in-house talent with the technical expertise required to build, train, and maintain AI models. Without a knowledgeable team or experienced partner, companies risk creating AI solutions that are difficult to scale or even deploy.
- Cost and resource allocation: AI projects can be resource-intensive, requiring significant investment in hardware, software, and human resources. For companies new to AI, costs can quickly escalate. Our company mitigates these risks by focusing on efficient, scalable solutions that maximize ROI. To better plan and manage these costs, consider using an AI cost calculator.
- No alignment with goals: AI initiatives will not show any ROI as long as they are not in line with your bigger business goals.

Misalignment with Business Goals
Too often, AI projects are launched without a clear connection to business objectives.
While it’s tempting to explore AI capabilities for their novelty, unless there’s a clear, measurable goal, the project can end up as a costly distraction. Many companies find themselves stuck in what’s known as “POC purgatory”—they successfully develop proofs of concept, but these experiments never transition to real-world applications.
This misalignment stems from a disconnect between technical teams and business leaders. AI teams may focus on model performance or accuracy, while executives care about ROI and operational efficiency.
In our experience, the solution to this lies in bridging this gap by aligning AI initiatives with core business drivers from day one. Instead of “doing AI for AI’s sake,” Tribe AI helps clients target specific outcomes—whether it’s improving customer retention, boosting productivity, or cutting costs.
This focus ensures that every AI project serves a strategic purpose, moving the needle on business goals rather than gathering dust in a lab.
AI Adoption and Underestimation of Change Management Needs
AI doesn’t just disrupt technology; it disrupts people and processes, too. Many leaders underestimate the level of change management required for successful AI adoption.
Deploying AI requires more than just technical integration—it involves retraining employees, adjusting workflows, and fostering a culture of adaptability. Here are some of the most common human-based roadblocks to AI adoption.
- Resistance to change: Employees accustomed to traditional workflows may resist new AI-driven processes, fearing job displacement or unfamiliarity with the technology. AI consultants and AI adoption agencies work closely with organizations to ease this transition, offering support to simplify AI adoption and integrating AI tools into daily routines.
- Ongoing maintenance and updates: AI models aren’t static. They require constant monitoring, updates, and retraining to remain effective. Without a long-term plan for model maintenance, companies risk their AI applications becoming outdated or ineffective. Setting up infrastructure for ongoing maintenance, ensuring the model evolves alongside the business is the way to go here.
- Cross-Functional collaboration: Successful AI deployment requires input and collaboration from multiple departments, including IT, operations, and customer support. Siloed teams can slow down or even derail an AI project. Companies should foster cross-functional collaboration, ensuring alignment across departments to make AI integration as seamless as possible.
AI adoption is as much about people as it is about technology. Clients can avoid the trap of failed implementations and build AI systems that not only work technically but also fit into the organization’s culture.
Key Areas of AI Value Creation
Tribe AI’s expertise spans several key areas, helping organizations turn AI from a novel technology into a true business driver. Here’s how we create real value across core operational areas.
Knowledge Process Automation and Information Access
For industries drowning in data, knowledge process automation with AI is like flipping on a light switch in a dark room. The goal is to create AI solutions that streamline information access, allowing companies to find insights quickly without sifting through endless files or datasets. This approach transforms data-heavy tasks—from market research to regulatory compliance—making it easy for teams to access and act on critical information.
Enhanced Software Development with AI Tools
In software development, where time and quality go hand in hand, AI is reshaping what’s possible. We help companies leverage advanced tools to speed up coding and improve code quality.
- Increased coding efficiency and quality: AI tools such as automated code review and error-checking can catch issues early, reducing the need for extensive manual debugging. This leads to cleaner code and faster release cycles, freeing developers to focus on complex problems instead of repetitive tasks. Developers can utilize AI techniques for developers to enhance productivity and code quality.
- GitHub copilot and developer experience: By integrating tools like GitHub Copilot, AI consultants enhance the developer experience, allowing coders to receive suggestions and code completions in real-time. This integration means developers spend less time on syntax and more on innovation. With the reduced cognitive load, developers report higher satisfaction and productivity, boosting morale and project velocity.
AI in Customer Care and Support Automation
Customer support can be costly and challenging to scale, especially for global operations. AI offers a solution by automating routine interactions and providing instant responses.
24/7 support, cost reduction, and global reach: AI-powered chatbots and support agents allow companies to provide around-the-clock customer service without adding extra shifts or staff. These bots can handle repetitive inquiries, resolve common issues, and even escalate more complex problems to human agents when needed. The result? Significant cost savings and improved response times, all while enhancing the customer experience with instant, accurate responses. Businesses can improve customer care with AI by implementing these solutions.
Sales and Marketing Optimization
AI’s predictive power can transform how companies approach sales and marketing.
Targeted campaigns and lead scoring: Outcome-focused AI solutions use data to predict customer behavior, helping companies to target their campaigns with pinpoint accuracy. Whether it’s lead scoring or churn prediction, AI-driven insights allow sales teams to prioritize high-value leads, improving conversion rates and driving revenue growth. This targeted approach maximizes every dollar spent, making marketing efforts more effective. For strategies on enhancing efficiency, consider exploring AI in sales efficiency.
Legal Automation and Compliance Monitoring
In legal and compliance-heavy industries, where accuracy is non-negotiable, AI can bring automation that saves time and reduces human error.
Streamlined document review and monitoring: AI-driven legal solutions can automatically analyze and categorize documents, flagging relevant clauses and compliance risks. This capability is a game-changer for legal teams who no longer need to comb through contracts manually. Instead, AI helps spot issues instantly, reducing review times and ensuring that no crucial detail is overlooked.

How to Tackle Technical and Operational Barriers in AI
Implementing AI comes with challenges that can make even the most promising projects stall. For companies to get past these barriers, they need a practical approach that considers data, cost, skills, and integration. Here’s how Tribe AI tackles the common hurdles that stand in the way of successful AI deployment.
The Importance of Data Readiness and Quality
AI is only as good as the data it’s trained on. Yet, many companies struggle with messy, incomplete, or siloed data that hampers AI’s effectiveness. Before any AI system can deliver results, the data feeding it has to be reliable, structured, and readily available.
- Cleaning and organizing data: Assessing data quality as early as possible is paramount, in order to identify data gaps and inconsistencies. This preparation prevents the “garbage in, garbage out” problem, where flawed data leads to unreliable AI outputs. Building a data-driven culture with AI ensures that your AI initiatives have a solid foundation.
- Breaking down silos: Often, data lives in separate silos, which can complicate access. We work with companies to integrate these data sources, ensuring that our AI systems draw from the most comprehensive dataset possible.
AI Models Optimization for Cost Efficiency and ROI
AI projects can be expensive, especially if they aren’t set up to generate measurable ROI. Many companies invest heavily in AI only to find that the costs spiral out of control without the returns they expected. Tribe AI focuses on cost-efficient models that deliver value from day one.
- Avoiding runaway costs: Generative AI models come with variable costs, especially if they’re processing large volumes of data. Models that are efficient and scalable need to be emphasized, so companies aren’t burdened with unexpected expenses.
- Focusing on ROI from the start: Consultants like Tribe AI align each project with specific business goals, whether it’s reducing customer service costs or improving sales forecasting accuracy. This focus ensures that every model contributes directly to the bottom line. Addressing common AI solution challenges can help in optimizing models for better ROI.
Addressing Talent Gaps and Skill Deficiencies
AI expertise is scarce, and many companies struggle to find skilled professionals who understand both AI technology and its practical applications. Without the right talent, even the best AI models can fail to take off.
- Providing access to top talent network: Companies should work on connecting with experts who have real-world AI experience. These are professionals who know how to build, test, and refine AI models for business contexts, minimizing trial and error.
- Training existing teams: AI isn’t a one-person job. For example, Tribe AI often works alongside in-house teams, upskilling employees to manage and maintain AI systems. This collaborative approach builds internal expertise, reducing reliance on external consultants in the long run.
The Complexity of Integrating AI into Existing Systems
Integrating AI into legacy systems can be like fitting a square peg into a round hole. Existing technology stacks and workflows are rarely built with AI in mind, making it challenging to add AI capabilities without disrupting operations.
- Creating compatibility with legacy systems: Making new AI solutions “play nice” with older systems is the name of the game. This might mean developing custom APIs or building data pipelines that allow seamless data flow between AI models and other software. For finance sectors, understanding how to integrate AI in finance systems can be particularly crucial.
- Minimizing disruption: By focusing on incremental integration, companies can avoid the “big bang” approach. This phased rollout minimizes disruption to daily operations, allowing the AI to start small, prove its value, and expand gradually. Embracing digital transformation with AI requires careful planning to integrate new technologies smoothly.
The Current and Future Potential of Generative AI
Generative AI has already made significant strides in helping businesses streamline operations, enhance customer interactions, and tackle complex problem-solving. But its capabilities are still evolving. Here’s where we stand with generative AI today—and where it’s headed.
Core Capabilities of Generative AI Today
Generative AI’s functionality is rapidly expanding, but its core strengths focus on three primary areas that are already providing real value.
- Language Processing and Natural Language Generation: Generative AI models like GPT-4 and Perplexity excel in understanding and generating human-like text. These capabilities have transformed customer service with AI-driven chatbots that can answer questions, offer support, and even engage in conversations that feel natural. Businesses are using these models to handle inquiries, reduce wait times, and create seamless interactions at scale.
- Information synthesis for quick insights: Generative AI can take vast amounts of data and quickly pull out the key points. This is a game-changer for sectors like healthcare, finance, and legal, where critical information is buried in lengthy documents or complex datasets. AI models now help teams find insights faster, freeing up time and allowing employees to focus on higher-value tasks. For more on how AI is impacting sectors like healthcare, read about AI in healthcare.
- Creative and Adaptive Problem-Solving Capabilities: Generative AI adapts to tasks ranging from content creation to code suggestions. In software development, AI tools like GitHub Copilot offer code completions, improving efficiency and reducing frustration for developers. It’s creative problem-solving at its best—AI suggesting new solutions or completing complex tasks that once required hands-on human oversight.
Future GenAI Innovations
While generative AI’s current abilities are impressive, the technology is on the cusp of several groundbreaking advancements.
- Autonomous task completion: Soon, AI won’t just answer questions—it’ll handle entire projects with minimal human input. Imagine a scenario where AI could manage research, plan strategies, or even coordinate team tasks, all autonomously. This shift could save countless hours for knowledge workers and boost productivity across industries. Exploring various Generative AI use cases can provide insights into these advancements.
- Adaptive learning and skill development: Generative AI is becoming more adaptable, capable of learning new tasks and skills over time. This means AI will be able to handle increasingly complex tasks in fields like personalized education and employee training, helping individuals grow and adapt alongside the AI. Companies can expect more tailored, ongoing support from AI that "learns" with them, from them.
- Collaborative AI and human problem solving: The future of generative AI isn’t replacing people—it’s working alongside them. AI and humans will collaborate on complex challenges, from scientific research to innovative product development. This partnership will allow businesses to tackle big problems faster, combining AI’s data processing power with human intuition and creativity. For businesses looking to enhance sales, understanding how to boost sales with generative AI can be particularly beneficial.
GenAI in Software Development: Stats and Trends
GitHub’s CEO, Thomas Dohmke, said that "Sooner than later, 80% of the code is going to be written by Copilot. And that doesn't mean the developer is going to be replaced."
This points to the general mood when it comes to acceptance of AI in software development, an area particularly benefiting from GenAI development. And numbers do point in that direction.
Comparing GitHub’s study from 2022 to the more recent one from 2024, the latter says that 90% of developers feel more fulfilled with their job and 95% enjoyed coding more, compared to 60%-75% of developers in 2022.
Google’s own research from 2024 pointed to assisted code reviews leading to a 37% acceptance rate for suggestions, compared to 25-34% in 2022.
Generative AI is no longer a novelty; it’s a tool—a particular kind of AI application, even—with proven capabilities and enormous potential.
As businesses look to the future, the focus will be on expanding these abilities to improve efficiency, deepen insights, and foster seamless collaboration between AI and human teams.
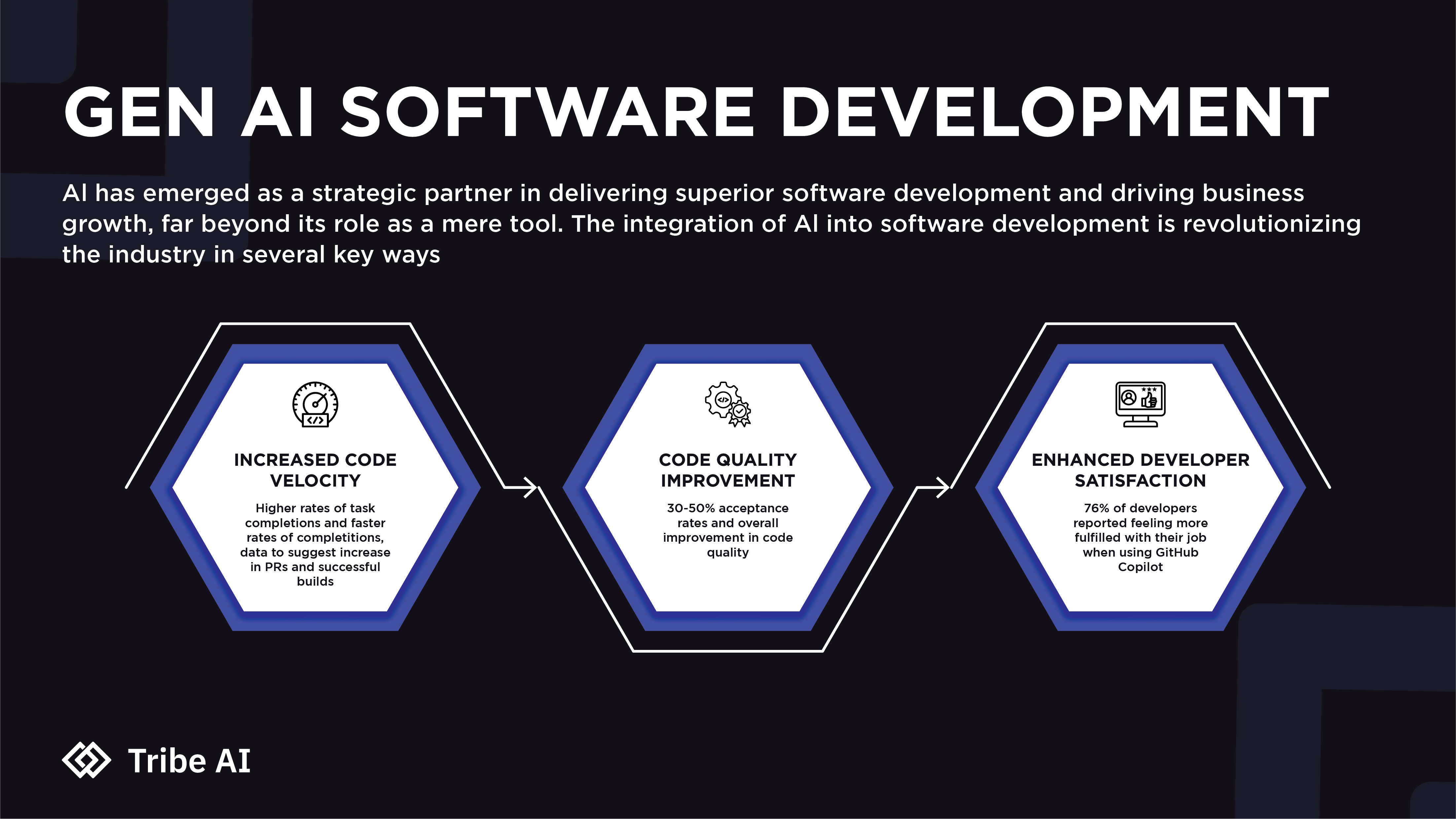
Real-World Case Studies: AI’s Proven Business Impact
Tribe AI has helped various organizations implement AI solutions that don’t just perform but also produce tangible business outcomes. Here are some examples that show how AI, when thoughtfully deployed, drives impact.
How Klarna Streamlined Customer Service with AI
Klarna, a global payments provider, needed to manage a high volume of customer inquiries quickly and efficiently. Using OpenAI, Klarna automated large parts of its customer service operations, achieving remarkable results.
Cost savings and improved customer satisfaction: Klarna’s AI solution handled millions of customer conversations, reducing the need for human agents by over 50%.
The result? Reduced operational costs and a substantial improvement in response times. AI handled routine questions in seconds, freeing human agents to manage complex issues. Specifically, Klarna managed to achieve in the first month of AI deployment:
- Handled 2.3M conversations—2/3 of Klarna's customer service chats (Equivalent to the workload of 700 full-time human agents)
- Reduced resolution time from 11 minutes to 2, while keeping same customer satisfaction scores as human agents
- Estimated to drive a $40 million USD profit improvement
- Revenue per employee surged by 73% in the last 12 months
This strategy not only saved money but also improved customer satisfaction, as customers experienced shorter wait times and faster resolutions.
Voice-Enabled Logging for MyFitnessPal
MyFitnessPal wanted to simplify the experience of logging meals for its 200+ million users. Logging food entries can feel like a chore, and they saw AI as a solution to make it less of one.
Enhancing user experience and driving engagement: Tribe AI helped MyFitnessPal introduce voice-enabled logging for their Premium users, allowing them to record meals by speaking as if they were talking to a friend instead of typing in and categorizing every ingredient. The voice interface made tracking food intake simpler and faster, which drove user engagement. For many users, this new feature felt intuitive and encouraged more frequent app usage. By reducing friction in the logging process, MyFitnessPal saw more users actively engaging with the app, helping the brand reinforce its value and improve retention.
Corporate Learning Transformation with AI at Litmos
Litmos, an online learning platform, wanted to improve learning experiences by making them more personalized and engaging, by developing 1-on-1 tutoring. With Tribe AI’s support, they developed an AI-powered assistant to help deliver content tailored to each learner’s needs.
Personalized learning and increased user adoption: The AI assistant in Litmos helps learners navigate content, answer questions, and find relevant materials. Tribe started building a PoC for Q&A, course recommendations, and agents for administrators in order to introduce users to chat interfaces. Users now experience a more tailored learning journey, which makes courses feel more relevant and engaging. The personalized experience has led to higher rates of course completion and platform adoption, as learners find they get more value from each session.
AI-Powered Customer Support for Government and Enterprise
Government agencies and large enterprises often face a flood of service requests, many of which are repetitive. Tribe AI worked with government and enterprise clients to create AI chatbots and routing systems that handle such requests more efficiently.
Reducing Costs and Enhancing Accessibility: By automating responses to common questions, these AI-driven solutions cut down on wait times and made information more accessible to the public. In one project, a government agency used an AI chatbot to handle 311 requests, providing around-the-clock support and routing more complex requests to the right departments. This approach saved costs on personnel and provided faster service to citizens.
Another case study illustrating AI's impact is how an insurance company uses ML to optimize pricing. It shows how machine learning can lead to better pricing strategies and improved profitability.
Each of these case studies underscores a key point: AI is not about flashy tools but real results. Tribe AI’s approach combines the right technology with an understanding of business priorities, turning AI potential into impactful outcomes.
Take a look at Tribe AI case studies for more use cases and companies Tribe AI helped build AI solutions that solved their business challenges.
AI Pricing Models and Market Trends
As AI adoption accelerates, companies are exploring diverse pricing models to make AI accessible while aligning costs with real business value. Here’s how AI pricing is evolving and what it means for businesses looking to adopt AI solutions.
The Rise of Subscription and User-Based Pricing Models
Many AI platforms now follow the familiar subscription or user-based pricing models. This approach charges customers based on either the number of users or a flat monthly fee, providing predictability for budgeting. These models cater to companies looking for straightforward solutions without high variability in costs.
For example, tools like OpenAI’s ChatGPT offer tiered subscriptions that unlock different levels of capability, allowing businesses to pay only for what they need. Subscription and user-based pricing have become especially popular for companies using AI as a productivity “copilot,” where predictable monthly costs make AI an accessible tool for a range of applications.
Hybrid and Success-Based Pricing Examples
Some AI providers are moving towards hybrid models, which combine a fixed base fee with success-based pricing. In these models, clients pay a baseline cost but are also charged based on the performance or outcome the AI achieves. This pricing structure aligns incentives, as providers are motivated to deliver impactful results, and clients are assured that they’re paying for real value.
Success-based pricing is particularly appealing in sectors where AI’s effectiveness can be directly measured, such as customer support and sales. For instance, Intercom’s AI chatbot, Fin, charges $0.99 per successful support resolution, directly tying cost to outcome. This model is gaining traction as it provides a fairer cost structure, reflecting actual ROI rather than upfront investment alone.
Real-World Examples of Success-Based Pricing
Success-based pricing is a model that has found practical applications across a range of AI services. Microsoft’s AI-powered security copilot charges clients on a “pay-as-you-go” basis, allowing them to scale their usage according to need. Similarly, Salesforce’s Agentforce uses a per-interaction cost, with discounts for higher volumes, giving companies flexible pricing that aligns with their engagement levels.
Sumo Logic offers success-based pricing by allowing users to ingest and index data at no cost, only charging when data is queried. This approach ensures that customers only pay when they gain actual insights from the data, a big draw for companies managing fluctuating AI needs. These models provide companies with cost control and ensure that every dollar spent on AI contributes to their goals.

Recommendations for Business Leaders Considering AI
AI offers significant potential, but jumping in without a clear roadmap can lead to costly missteps. Business leaders should take a thoughtful, results-focused approach to ensure AI investments pay off.
Identify High-Impact Areas for AI Deployment
Not every process benefits equally from AI, so start by identifying areas where it will create real value. AI shines in data-heavy, repetitive tasks—think customer support, compliance checks, or inventory management.
Ask yourself: What tasks are slowing us down? Where do we lose the most time to manual work? These “pain points” are likely the best candidates for AI solutions.
By focusing on high-impact areas, you ensure AI is solving problems that matter. For example, if customer service is a bottleneck, an AI chatbot could reduce wait times and costs simultaneously. Prioritize use cases that provide immediate returns, building momentum and confidence in AI’s potential.
Align AI Projects with Clear Business Metrics
A common mistake with AI is starting projects without measurable goals. To avoid this, tie each AI initiative to specific business metrics. Whether it’s reducing operational costs, improving customer satisfaction, or boosting productivity, every AI project should have a metric to track its success.
For instance, if an AI tool is introduced to optimize sales, define targets for conversion rates or customer retention. Clear goals help avoid the “endless experiment” trap, ensuring AI projects move out of proof-of-concept and start contributing to the business.
Secure Cross-Functional Buy-In for AI Initiatives
AI projects often require buy-in from multiple departments, not just IT. For AI to succeed, teams across marketing, finance, customer support, and HR need to understand and support its role. Open conversations about AI’s impact can address potential concerns, from job changes to shifts in daily workflows.
Bringing cross-functional teams on board can also highlight hidden opportunities for AI. For example, finance might see value in using AI for risk assessment, while HR could benefit from AI-powered resume screening. By getting everyone on the same page, you foster a collaborative approach that increases AI’s success rate.
Focus on Long-Term Maintenance and Iteration
AI isn’t a one-and-done solution. Models need regular updates and fine-tuning as business needs and data evolve. Assign a team or partner dedicated to maintaining the model, monitoring its performance, and adapting it to new trends or requirements.
A focus on iteration means AI grows alongside the business, staying relevant and effective over time. Instead of letting the model go stale, you’re investing in long-term adaptability, which ultimately delivers sustained value. Treating AI as an evolving tool rather than a static product helps maximize its potential while minimizing the risk of it becoming outdated.
Tribe AI’s Accelerated and Derisked AI Deployment Strategy
In AI, speed and precision are everything—in fact, they are almost the whole point. Tribe AI understands that companies need fast results without the constant risk of setbacks, which is why our strategy focuses on accelerating deployment while reducing risks.
Decreasing Time from Concept to Production
Most companies can’t afford to wait years to see the benefits of AI, especially as the market becomes more competitive. Tribe AI’s deployment strategy is designed to bring ideas from concept to production in months, not years.
This isn’t about rushing; it’s about streamlined processes that allow organizations to iterate quickly. By working through cycles of testing and refinement, Tribe AI keeps the project moving forward with fewer bottlenecks.
Using Top AI Talent to Deliver Results
Tribe AI boasts a network of AI experts who bring experience from top tech firms like Google and OpenAI. This level of expertise makes a critical difference, ensuring that each project is handled by specialists who understand both the technology and its practical applications.
When experienced professionals are driving the project, the risk of costly missteps drops significantly. Tribe AI’s approach connects companies to this high-caliber talent, giving them the resources to tackle even the most complex AI challenges.
Importance of Robust Prototyping and Iterative Development
AI deployment is rarely a “set-it-and-forget-it” process. Tribe AI prioritizes rapid prototyping and iterative development to test ideas in real-world scenarios before committing to full-scale implementation.
Through this approach, Tribe AI helps clients identify what works and what doesn’t early in the process, reducing the risk of large-scale failures down the road. This agile approach ensures that adjustments can be made as insights emerge, keeping the project aligned with business goals.
Ensuring Scalability from POC to Full Deployment
Proof of concept (POC) is a good start, but too many companies get stuck there. Tribe AI’s strategy doesn’t stop at proof of concept; instead, it emphasizes scalability right from the start. Tribe AI ensures that every solution can be scaled to meet full production needs, supporting long-term growth rather than remaining a short-lived experiment. Our focus on scalability saves clients from the “POC purgatory” that many organizations experience, moving beyond test runs to solutions that can evolve with the business.
Building Solutions Aligned with Real-World Constraints
Tribe AI understands that real-world constraints—budget limits, regulatory requirements, and technical feasibility—can’t be ignored. We design each solution with these factors in mind, ensuring alignment with both the company’s strategic objectives and operational realities. By respecting these constraints from the beginning, Tribe AI delivers solutions that are not only effective but sustainable. This way, clients avoid investing in technology that looks good on paper but falls short in practice.